LinkedIn Live Recap: Unpacking the European AI Act for Governance & Data Teams
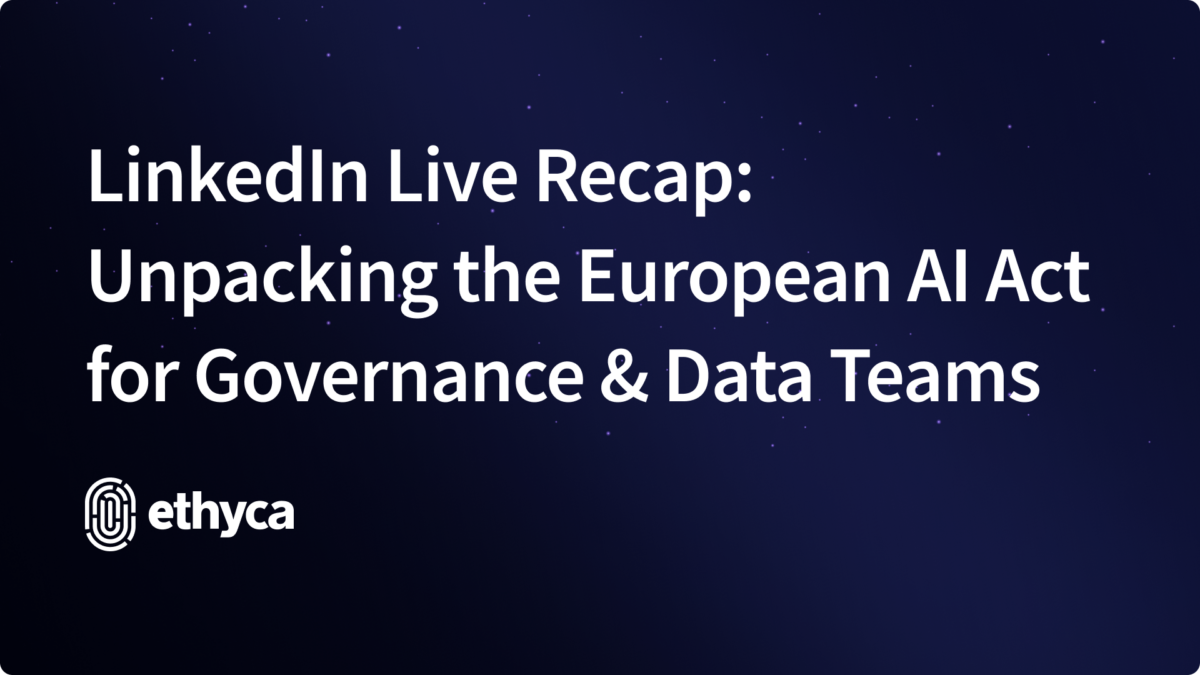
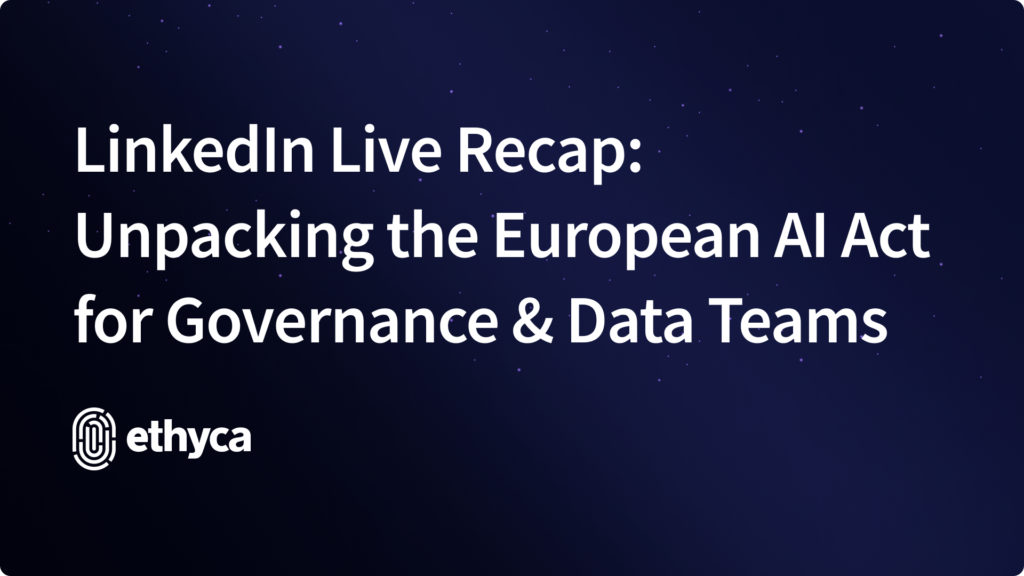
With the European Parliament’s landmark agreement on the Artificial Intelligence Act (AI Act) on December 8, 2023, our Ethyca CEO Cillian Kieran hosted a rapid-response LinkedIn Live about the technical requirements for governance, data, and engineering teams.
In his talk, Cillian covered how privacy and governance can be approached through an engineering perspective, what the main technical requirements of the EU AI Act are, focusing on risk management and data governance, and how engineers can implement solutions to those challenges.
Below is a recap of his main takeaways.
The EU AI Act divides AI systems into four risk thresholds
The EU AI Act categorizes AI systems into four different risk thresholds: low, limited, high-risk, and unacceptable risk. Each category level corresponds to the amount of regulation and oversight it’s subject to.
Low-risk AI systems
Low-risk, or minimal-risk models, have a low potential for creating risk or harm to EU subjects. These technologies include automated tools that don’t collect consumer data, such as spam filters. Low-risk AI systems have the least amount of regulations and oversight, if any, that they must follow.
Limited-risk AI systems
Limited-risk AI systems, like an automated chatbot, are subject to more transparency guidelines. For example, limited-risk systems require extensive documentation about how the AI system was trained. Users must also be notified that they are interacting with an automated tool and should be given enough information to make an informed choice on whether or not to use it.
High-risk AI systems
High-risk AI systems (the main focus of Cillian’s talk) are subject to strict regulations and guidelines. Although they are permitted on the market, these AI systems carry potentially high risks to users and must be heavily scrutinized before they’re deployed to the market.
Unacceptable-risk AI systems
Finally, unacceptable-risk AI systems are strictly forbidden in the EU, with a few exceptions. Examples of these banned practices of AI include the use of cognitive manipulation, individual predictive policing, and social scoring.
Cillian’s talk focused on how organizations can embed privacy into the development and deployment lifecycle of high-risk AI systems to mitigate potential risks and harms to users while complying with the AI Act.
High-risk models have 10 technical requirements
AI systems that are categorized as high-risk have 10 technical legal obligations that must be adhered to. Cillian briefly described them during his talk. They are:
- Quality management systems: Operators of AI must maintain high-quality documentation, policies, and record-keeping to ensure proper enforcement of policies.
- Conformity assessment: Organizations must perform conformity assessments to demonstrate that their AI systems are compliant through testing and inspection.
- Corrective action: An AI provider should withdraw their system from the market if they find a new risk, or if it doesn’t meet conformity standards.
- Risk management: continuously evaluating risk throughout the AI development and deployment lifecycle.
- Data governance: defining and enforcing policies in the AI development lifecycle and data processing lifecycle after it’s deployed.
- Technical documentation: build and maintain documentation before an AI system is deployed and starts processing user data.
- Record keeping: maintaining an audit trail of all the data processing that occurred, from collection to training to its potential outcomes and risks.
- Transparency: the obligation of providing notices to the user that they are interacting with an AI system and for what purpose.
- Human oversight: AI should be designed with humans to ensure accountability, humanity, trust, and transparency.
- Accuracy, robustness, and security: Organizations must maintain a high level of data accuracy so that the outcome is not based, and it has the right security controls in place.
Risk management and data governance require deep integration into the development and data processing lifecycle
After outlining the 10 technical requirements of high-risk systems, Cillian dove deeper into two technical requirements: risk management and data governance, and how to perform them in a modern, complex tech stack.
Risk management
Technical risk management involves the end-to-end identification, mitigation, and recording of risks in the AI development and deployment lifecycle. This is similar to the kinds of risk assessment used in broader governance, and the proactive approach of Privacy by Design.
To assess risk in complex, modern software development and data processing life cycles Cillian emphasizes that we need more context; We need to understand the purpose for building the system in the software development process, as well as the purpose of using the AI system for your organization in the data processing life cycle.
You would then need to know what data is being handled in those systems before you can start enforcing policy checks in the software and data processing lifecycle to ensure the appropriate data is in the appropriate system for the appropriate purposes. You’d also need an audit trail to prove compliance to regulators.
Data governance
Technical data governance involves defining and applying policies that the organization has committed to based on regulatory requirements and internal policies. Data governance also involves enforcing those policies on business processes, like data engineering pipelines and data sets.
To enforce and govern policies on AI systems, you’d need the same thing for technical risk management: the purposes in relation to the software development and data processing lifecycle, as well as what data is being handled.
Additionally, you’d need to build context throughout your systems, i.e. purpose, data, subject, etc, and combine it with users’ privacy rights, such as the legal basis of processing, before you can start enforcing policy checks for the software development and data processing lifecycle.
Governance and data engineering teams need an ontology to apply uniform labeling
Finally, Cillian goes through how we can actually do this. The answer: with an ontology, or a taxonomy that provides a uniform way to define and label the types of data being handled, the purposes of using that data, and the potential risks.
With a shared understanding of what these labels are across your organization, you’ll be able to label the data accurately so it doesn’t flow into the wrong models or systems for the wrong purposes. As Cillian said, “Labeling is a core, contextual capability of great governance from an AI perspective.”
With the right language, you can enforce these conditions into the software development and data processing lifecycle. Once the data is properly labeled, you and your governance teams can be alerted to potential risks, and proactively mitigate them by enforcing the policy.
Embed AI Act policies into your software and data processing lifecycles with Ethyca
You can find or download Cillian’s slides here.
If you have any more questions about the EU AI Act, Cillian’s LinkedIn live, or what your business needs to do to comply, schedule a meeting with one of our privacy deployment strategists today.